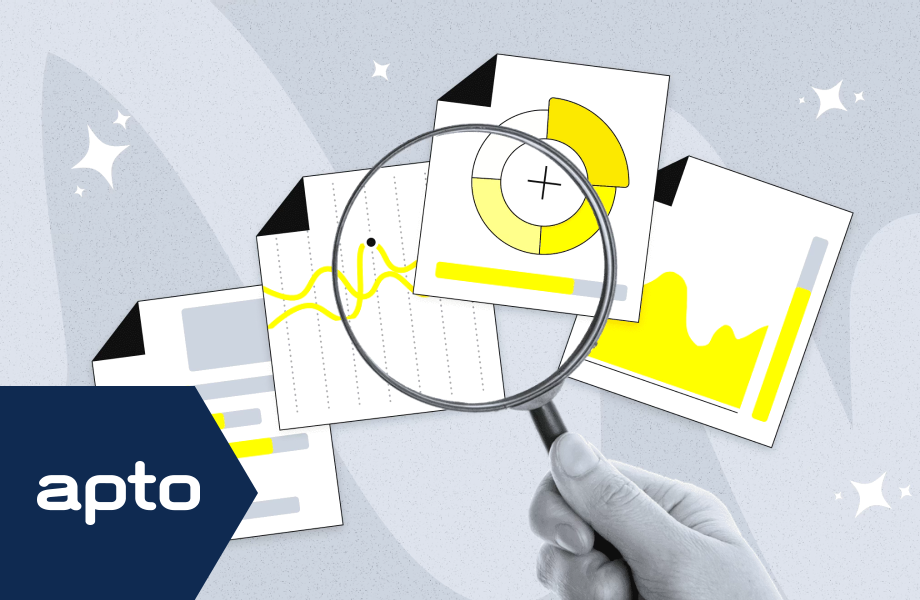
Data is the lifeblood of most organisations. Yet, effectively leveraging data requires a clear understanding of each step in the data pipeline – from collection to analytics. By exploring four key stages in the data journey: Data Sources, Data Processing, Data Storage, and Data Consumption – organisations can better understand their data, enhance operational efficiency, and make informed decisions that drive meaningful outcomes. This blog dives into why understanding each stage is critical and how it helps businesses unlock the true potential of their data.
Data Sources: Knowing Where Your Data Comes From
Understanding the origins of your data is the first critical step. Data sources are the points where data is generated, collated, or captured. In a business context, data can come from a vast array of sources, from employee laptops and mobile devices to building access card transactions and specialised hardware in sector-specific environments, like X-ray machines in airports or credit card readers in financial institutions.
Each data source provides unique insights, helping organisations build a comprehensive data landscape – a map of where and how data is generated across the organisation. This comprehensive view allows companies to:
- Identify Key Data Streams: Knowing the origin of data points can clarify how different sources contribute to the larger data ecosystem.
- Optimise Data Collection: By understanding data origins, companies can streamline data collection efforts to ensure data relevance and quality.
- Enable Targeted Processing: With clear insight into the nature of data sources, organisations can tailor their data processing techniques to specific data types.
By building a solid foundation within data sources, businesses can make informed decisions about data handling and management, setting the stage for efficient data processing and analysis.
Data Processing: Transforming Raw Data into Usable Information
Once data is collected, it needs to be processed, this can include aggregation, standardisation, and transformation of data into a consistent format. For many organisations, this involves log aggregation tools like Splunk, which capture data from various sources for analysis. Standardisation is crucial across the pipeline ensuring data remains consistent, usable, and free from vendor lock-in.
Effective data processing benefits include:
- Enhanced Data Quality: Standardising and cleaning data makes it reliable for analysis, avoiding discrepancies that may lead to inaccurate insights.
- Interoperability: A well-processed dataset allows companies to switch between vendors or integrate with new platforms seamlessly.
- Cost Efficiency: Streamlined data processing minimises redundancy, which can help reduce costs associated with high-cost platforms like Splunk.
For instance, standardising data formats allows companies to avoid being tied to a single data aggregation provider, empowering them to make flexible decisions about data ingestion and future-proofing their data pipeline.
Data Storage: Choosing the Right Solutions for Long-Term Data Management
After data is processed, it must be stored in a way that aligns with the organisation’s strategic goals. Data storage involves choosing the right platforms, managing costs, and determining retention policies. Effective data storage strategy prevents unnecessary duplication and ensures that data is accessible when needed.
Key considerations in data storage include:
- Cost Management: Understanding where and how data is stored can prevent unnecessary expenditures. For example, storing data both in Splunk with long retention policies and in a separate data lake can be extremely costly.
- Data Accessibility: Choosing the appropriate storage solution for each data type allows organisations to quickly retrieve and analyse relevant information.
- Compliance and Governance: Effective storage strategies ensure data meets regulatory and compliance requirements, reducing risk and enhancing orginisational data governance.
An understanding of data storage helps businesses eliminate redundant storage costs and optimise data use, keeping resources focused on actionable insights rather than data excess.
Data Consumption: Making Insights Meaningful Through Effective Analysis
Data consumption is the point where stored data is analysed and insights are derived to inform business decisions. In this stage, organisations determine how, who, and why data is used, ensuring that analytics drive value. In data-driven organisations, this stage is typically focused on analytics and decision-making processes that directly support business objectives.
Important aspects of data consumption include:
- Value-Driven Analytics: Aligning data consumption with specific business needs, such as monitoring passenger throughput in airports, this ensures insights are relevant and actionable. A SIEM is another example.
- User Accessibility: Understanding who needs access to what data helps tailor access levels and user interfaces to optimise and target the analytics experience.
- Cost and Resource Efficiency: Data-driven management is only valuable if the insights derived justify the cost and resources allocated to data analysis.
The value of data-driven management is limitless for an organisation, by modeling data points against time and business context you are enabling precise and valuable insights. Some key examples include modeling normal workplace behaviour to holistically model threat intelligence, mapping data ingest against time to understand growth and budget, and simulating through put to understand common trends within your business such as seasonal demand – this allows you to make an informed decision about supply.
Without meaningful data consumption, data collection and storage become futile exercises. Understanding this stage helps organisations focus their efforts on generating insights that offer tangible benefits, maximising the return on investment from their data pipeline.
The Role of Data Governance in Data Understanding
Throughout each stage of the data pipeline, data governance plays a pivotal role. Effective data governance policies ensure that data is collected, stored, and used in compliance with regulatory standards, protecting the organisation and its stakeholders. From ensuring data quality and consistency to enforcing access controls and retention policies, data governance underpins the entire data ecosystem, reinforcing transparency and accountability at each stage.
Final Thoughts: Building a Data-Informed Future
Understanding your data pipeline – from sources through to consumption – is fundamental in leveraging data for strategic advantage. By comprehensively understanding data sources, processing methods, storage options, and consumption practices, businesses can build a robust, cost-effective, and insightful data ecosystem.
Investing in understanding data not only enables better decision-making but also drives continuous improvement across operations, ultimately ensuring that organisations remain agile, data-informed, and competitive in an ever-evolving landscape.
-
20 January 2025
Data Pipelining and How it can benefit the NHS
-
7 January 2025
What is Operate? And Why Do You Need It?
-
5 December 2024
Onboarding Azure Data into Splunk
See how we can build your digital capability,
call us on +44(0)845 226 3351 or send us an email…